整合臨床及電腦斷層影像特徵預測乳癌前導性化療的療效
整合臨床及電腦斷層影像特徵預測乳癌前導性化療的療效
根據世界衛生組織統計,乳癌目前是女性發生率最高的癌症,其發病後造成的致死率也相當高,乳癌的早期治療方式會在手術切除之前給予患者前導性治療,包括:化學治療、標靶治療及免疫治療等,這些治療已被證實可幫助縮小腫瘤,並且提高患者治療的預後。本研究團隊整理高醫體系過去十年的乳癌患者資料,發現少部分患者(約17.2 %)經過前導性治療後,其腫瘤組織切除後顯微鏡下完全看不到殘存的癌細胞,然而大部分患者(約82.8 %)經過治療後腫瘤區域仍有殘存的癌細胞。
由於乳癌患者的臨床特性不同,例如:臨床分期、乳癌種類(賀爾蒙接受器)及細胞型態等差異,以及乳癌病灶在顯影電腦斷層上呈現不同,例如:腫瘤大小、形狀、顯影程度及肉眼無法分辨的紋理變化等,可能會影響患者前導性治療的療效,因此本研究整合臨床特性及影像特徵,透過人工智慧機器學習的方式成功建立出準確的療效預測模型,結果發現「整合式模型」的預測準確性(87%)比過去所使用的「臨床模型」(69 %)及「影像模型」(78 %)的預測準確度來得高。因此,未來將可透過該人工智慧模型預測患者之療效,有助於臨床醫師能更準確地評估患者的治療策略,提早調整治療方案來改善患者的治療預後。
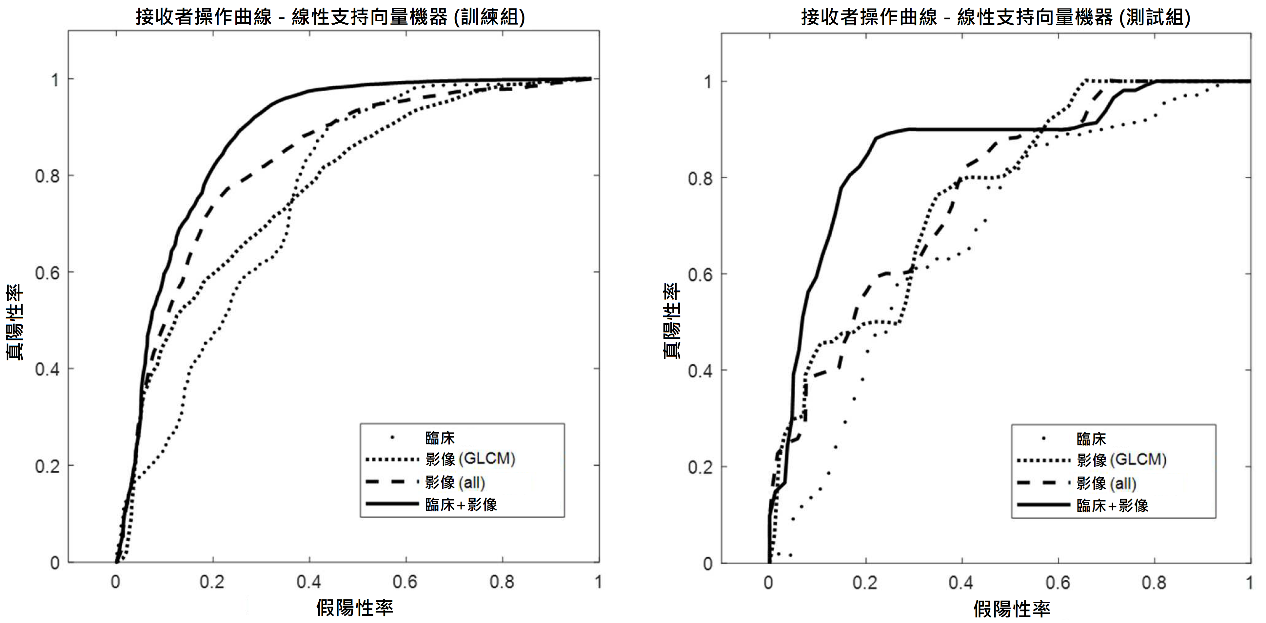
[左圖] 訓練組之臨床、影像及整合(臨床+影像)預測模型的接收者操作曲線圖。
[右圖] 測試組之臨床、影像及整合(臨床+影像)預測模型的接收者操作曲線圖。
應用與亮點:
1. 整合臨床及電腦斷層影像特徵有助於提升患者經前導性治療療效的預測準確性。
2. 患者的乳癌種類(賀爾蒙接受器)、腫瘤形狀與大小,以及影像上腫瘤細微的紋理變化,在人工智慧預測模型上扮演重要的角色。
【研究團隊】
團隊成員:蔡蕙怡、蔡宗育、吳佳蕙、鍾維軒、王若璟、許瑞昇、侯明鋒、周銘鐘
代表單位:高雄醫學大學/大數據研究中心、高雄醫學大學/醫學影像暨放射科學系、高醫附院/影像醫學部
團隊簡介:本團隊致力於結合人工智慧及醫學影像分析來提升醫療品質
研究聯繫Email:mcchou@kmu.edu.tw
【論文資訊】
論文出處: Tsai H-Y, Tsai T-Y, Wu C-H, Chung W-S, Wang J-C, Hsu J-S, Hou M-F, Chou M-C. Integration of Clinical and CT-Based Radiomic Features for Pretreatment Prediction of Pathologic Complete Response to Neoadjuvant Systemic Therapy in Breast Cancer. Cancers. 2022; 14(24):6261.
全文下載:https://doi.org/10.3390/cancers14246261
Integration of clinical and CT-based radiomic features for pretreatment prediction of pathologic complete response to neoadjuvant systemic therapy in breast cancer
Integration of clinical and CT-based radiomic features for pretreatment prediction of pathologic complete response to neoadjuvant systemic therapy in breast cancer
According to the statistics of the World Health Organization, breast cancer is currently the cancer with the highest incidence rate in women, and its fatality rate is also quite high. In routine practice, the early treatment of breast cancer is neoadjuvant systemic therapy before surgical resection, such as chemotherapy, targeted therapy, immunotherapy, etc. These treatments can help downsize tumors and improve the prognosis of those patients being treated. Therefore, the present study collected the data of breast cancer patients from the Kaohsiung Medical University affiliated hospitals in the past ten years, and found that only 17.2% patients had a pathologic complete response (pCR), but most patients (about 82.8%) still had in situ residual cancer cells after the neoadjuvant systemic therapy.
Breast cancer patients had different clinical characteristics, such as clinical stage, type of breast cancer (hormone receptor) and cell type, and different imaging characteristics on contrast-enhanced computed tomography, such as tumor size, shape, contrast enhancement and textures. These variety of factors may together influence the treatment outcome of neoadjuvant systemic therapy. Therefore, the present study integrated both clinical and radiomic features to establish a more accurate prediction model for pCR using an artificial intelligence-based machine learning technique. The results demonstrated that the predictive accuracy of the “integration model” (87%) was significantly higher than that of the "clinical model" (69 %) and the "radiomics model" (78 %). Therefore, with the assistance of prediction model, it will be helpful for clinicians to more accurately evaluate the treatment outcome prior to the therapy, and to adjust the treatment regime for improving the prognosis in patients with breast cancers.
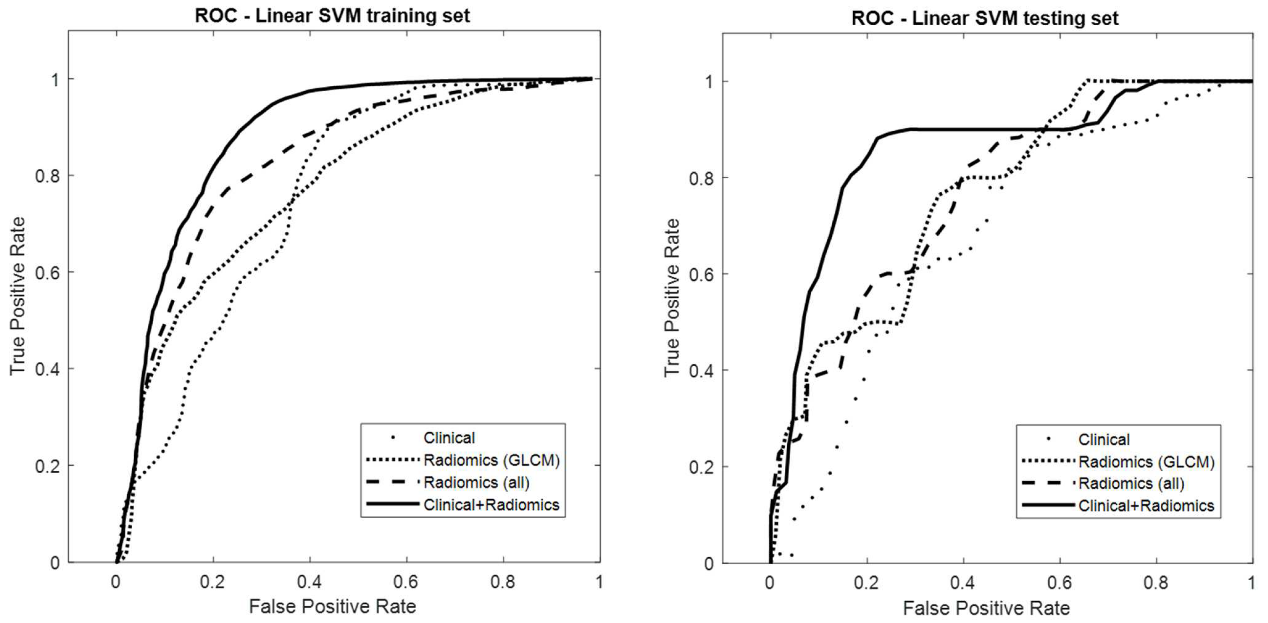
Left: The receiver operating characteristic curves of clinical, radiomics, and integration (clinical + radiomics) prediction models using training dataset.
Right: The receiver operating characteristic curves of clinical, radiomics, and integration (clinical + radiomics) prediction models using testing dataset.
Application and Highlights:
1. Integrating clinical and CT-based radiomic features can help improve the accuracy of predicting patients' response to pretreatment.
2. The type of breast cancer (hormone receptor), shape, size, and the subtle texture changes of the tumor played important roles in the prediction model.
Research Team Members:
Huei-Yi Tsai, Tsung-Yu Tsai, Chia-Hui Wu, Wei-Shiuan Chung, Jo-Ching Wang, Jui-Sheng Hsu, Ming-Feng Hou, and Ming-Chung Chou
Representative Department: Center for Big Data Research and Department of Medical Imaging and Radiological Sciences, Kaohsiung Medical University. Department of Medical Imaging, Kaohsiung Medical University Hospital.
Introduction of Research Team:
The team has been committed to combining artificial intelligence and medical image analysis to improve medical quality.
Contact Email: mcchou@kmu.edu.tw
Publication: Tsai H-Y, Tsai T-Y, Wu C-H, Chung W-S, Wang J-C, Hsu J-S, Hou M-F, Chou M-C. Integration of Clinical and CT-Based Radiomic Features for Pretreatment Prediction of Pathologic Complete Response to Neoadjuvant Systemic Therapy in Breast Cancer. Cancers. 2022; 14(24):6261.
Full-Text Article: https://doi.org/10.3390/cancers14246261