以人工智慧模組協助潛伏結核治療個人化處方選擇
以人工智慧模組協助潛伏結核治療個人化處方選擇
結核病(Tuberculosis disease)至今仍是全球死亡率最高之傳染疾病之一,在世界衛生組織努力之下,雖然全球結核病盛行率逐年緩慢下降,但其下降幅度距離所提出2035年終結結核病之願景仍有許多努力空間。當宿主吸入結核菌時,只有少數個案會造成肺部感染,宿主免疫狀況良好時可將肺部結核菌控制於不發病的平衡狀態,稱為潛伏結核感染(latent TB infection,LTBI),後續發病率約為5至10%。世衛組織估計全球約有四分之一人口為LTBI個案,且在結核病較低發生率國家,大多數的新發病個案來自於潛伏結核之再活化,而非再感染。因此,針對高發病風險之LTBI個案積進行極治療為世衛組織所提倡終結結核病之重要策略之一。LTBI的治療演變方向主要朝著有效、安全、短程、高完治率的處方進展。傳統為期長達9個月的單一Isoniazid(INH)處方(9H)因受限於治療時程長及肝毒性顯著而導致完治率不佳。後來rifapentine(RPT)的出現開啟了為期3個月共12劑次,每週服用一次依照體重調整之高劑量Isoniazid及rifapentine(15mg/kg,max 900mg/time)短程處方「速克伏」(3HP),此為目前服藥劑次最少之LTBI治療處方,大幅改善了病人的服藥順從性及完成治療率。可惜的是,使用3HP處方的個案中約有5-10%不等個案會出現全身性藥物不良反應(Systemic drug reaction,SDR)而造成超過50%個案因此中斷治療。SDR主要有兩種表型,第一種為類流感症候群(Flu-like syndrome),第二種則以全身性表徵包含休克、暈厥、蕁麻疹、喉頭及支氣管痙攣…等嚴重過敏相關症狀。但目前造成SDRs之原因仍未明。
本研究為多醫學中心之前瞻性觀察型研究,旨在探討服用3HP後是否出現SDR個案於服用3HP前及服藥後之週邊單核血球(peripheral blood mononuclear cell)之轉錄體基因(transcriptome gene)改變,進一步結合人工智慧AI 模組以發展病人在服用潛伏結核藥物處方前可以預測SDR 發生的快速基因檢測。本研究結合biological functional analysis和interpretable AI model 建立了臨床可使用且容易判讀的簡易基因模組(SHAP value cut-off = 0),其預測SDR結果之accuracy 為92.1%; sensitivity 為0.875 及specificity 為0.889。此預測模式將可於潛伏結核個案服藥前提供風險警示,讓臨床醫師可以事前評估在這些可能會出現SDRs 個案使用其它治療處方,增加個案接受預防性治療的安全度及治療成功率,並減少因嚴重藥物不良反應所增加的醫療負擔。
圖形摘要
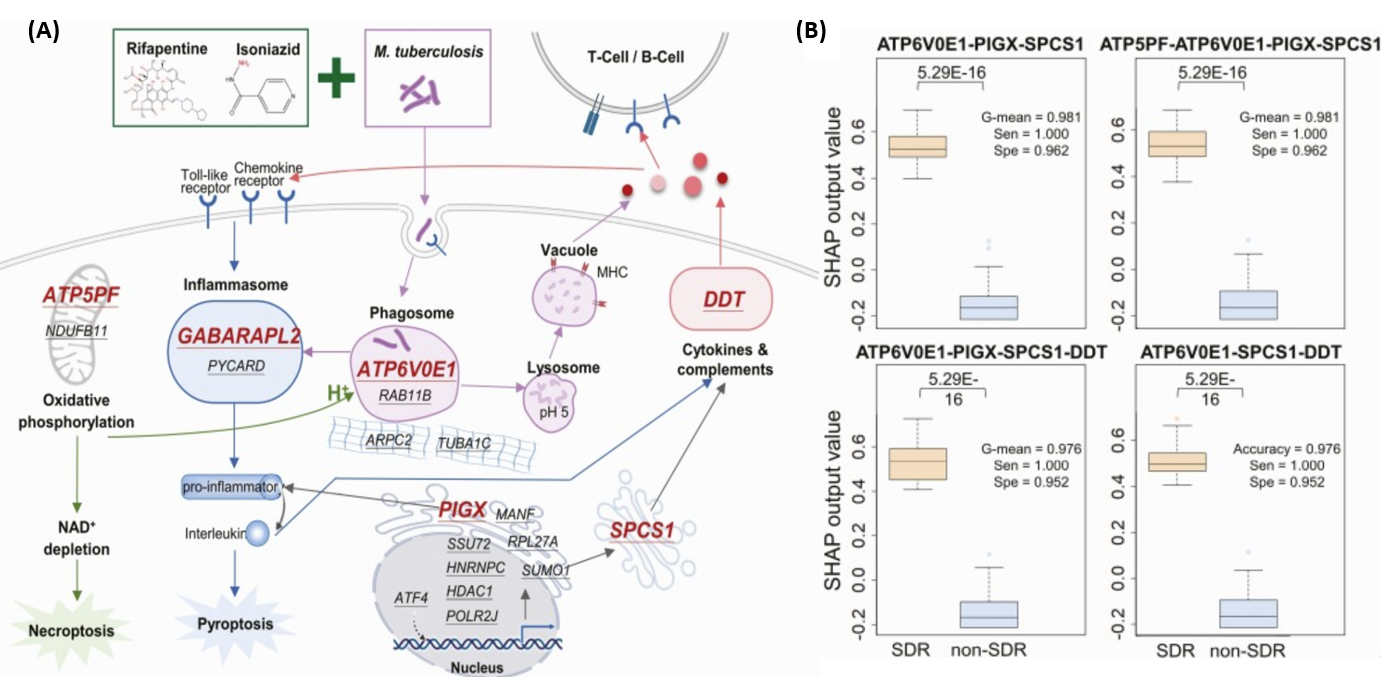
(A圖)顯示為3HP治療下與SDR發展相關的潛在基因的治療-生物標誌物路徑示意圖,其中有19個為潛在與SDR相關之轉錄體基因,經由基因表現篩選過後,共有6個轉錄體基因被篩選為建立預測模型之代表。
(B圖)盒狀圖顯示使用6個轉錄體基因轉置為SHAP值後,隨機建置模型後篩選出4個最佳表現模型。
應用與亮點:
亮點: 本研究以臨床副作用表型(phenotype)結合基因型(genotype),使用biological functional analysis及可解釋的AI模型(Interpretable AI model),建立臨床簡易基因預測模型。在潛伏結核治療前,使用基因檢測模型評估患者發生全身性不良反應的風險,從而制定更個人化的治療計劃
應用: 本研究模型結合AI技術和基因分析結合之方法學可應用於開發其他藥品嚴重副作用之風險預測模式。透過預測高風險患者,可以減少因藥物嚴重副作用造成的醫療支出與住院負擔,進而達到更有效的醫療資源分配。
【研究團隊】
團隊成員:
1.黃虹綾 Hung Ling Huang
https://wac.kmu.edu.tw/qur/profiles.php?id=990325
2.王振源 Jann Yuan Wang
https://www.ntuh.gov.tw/Med/Vcard.action?q_type=A04&q_itemCode=136
3.楊進木Jinn Moon Yang
https://dbt.nycu.edu.tw/faculty/yang-jinn-moon/
4.鄭孟軒 Meng Hsuan Cheng
https://wac.kmu.edu.tw/qur/profiles.php?id=880298
5.盧柏樑 Po Liang Lu
https://wac.kmu.edu.tw/qur/profiles.php?id=830166
6.鍾飲文 Inn Wen Chong
https://wac.kmu.edu.tw/qur/profiles.php?id=730138
7.李孟叡 Meng Rui Lee
https://www.hch.gov.tw/?aid=51&pid=3&page_name=detail&iid=5
8.李枝新 Chih Hsin Lee
https://glb2sys.tmu.edu.tw:8020/TchInfo_Public/TchInfo.aspx?f=Tch&key=MTAzMTA3&key1=
代表單位:高雄醫學大學附設醫院胸腔內科、台灣大學附設醫院胸腔內科、陽明交通大學生物科技學系、高雄醫學大學附設醫院感染內科、台北市立萬芳醫院胸腔內科
團隊簡介:本研究團隊成員有來自於高雄醫學大學附設醫院、台灣大學附設醫院、以及台北市立萬芳醫院,負責患者診治及臨床檢體收集的胸腔科及感染科醫師們及陽明交通大學生物科技學系藥物設計與系統生物實驗室楊進木教授團隊所組成,本研究團隊的中心思想為挑戰未知,開發可應用的臨床檢測及追求個人化的精準醫療。
研究聯繫Email:990325kmuh@gmail.com
【論文資訊】
論文出處:Clin Infect Dis. 2022 Sep 14;75(5):743-752. doi:10.1093/cid/ciac003.
全文下載: https://www.ncbi.nlm.nih.gov/pmc/articles/PMC9477448/
Using AI Models to Assist Personalized Prescription Selection for Latent Tuberculosis infection Treatment
Using AI Models to Assist Personalized Prescription Selection for Latent Tuberculosis infection Treatment
Tuberculosis (TB) remains one of the leading lethal infectious diseases worldwide, despite ongoing efforts by the World Health Organization (WHO). While the global prevalence of TB has gradually declined, there is still a significant gap in reaching the WHO's goal of End TB in 2035. Upon exposure to Mycobacterium tuberculosis, only a small number of individuals develop pulmonary infection. When the host’s immune system successfully controls the Mycobacterium tuberculosis without disease progression, the infection is termed latent tuberculosis infection (LTBI), with a subsequent TB disease activation risk of about 5–10%. WHO estimates that approximately one-quarter of the global population is infected with LTBI. In countries with low TB incidence, most new cases arise from the reactivation of LTBI rather than new infections. Consequently, preventive treatment for high-risk LTBI individuals is a critical strategy in WHO’s efforts to end tuberculosis.
The treatment for LTBI has evolved towards shorter, safer, and more effective regimens with higher completion rates. The traditional 9-month isoniazid monotherapy (9H) is limited by its long duration and significant hepatotoxicity, which negatively impacts treatment adherence. The introduction of a shorter 12-dose regimen of weekly isoniazid and rifapentine over 3 months (3HP), tailored by body weight (15 mg/kg, max 900 mg/time), has significantly improved treatment adherence and completion rates. This regimen, known as 3HP, is currently the treatment option with the fewest doses for LTBI. However, 5–10% of individuals on 3HP experience systemic drug reactions (SDR), with over 50% discontinuing treatment as a result. SDRs typically present in two phenotypes: (1) flu-like syndrome and (2) severe allergic reactions, such as shock, syncope, urticaria, and laryngeal or bronchial spasms. The underlying cause of SDRs remains unclear.
This multicenter prospective observational study aims to investigate transcriptomic changes in peripheral blood mononuclear cells (PBMCs) before and after 3HP treatment in individuals who develop SDRs and no SDRs. The study integrates an artificial intelligence (AI) model to develop a rapid genetic test that can predict SDR occurrence prior to LTBI treatment. By combining biological functional analysis with an interpretable AI model, we developed a simple gene-based predictive model (SHAP value cut-off = 0) that is both clinically applicable and easy to interpret. This model achieved an accuracy of 92.1%, with a sensitivity of 0.875 and a specificity of 0.889 in predicting SDR outcomes. The model provides a risk warning before treatment, enabling clinicians to assess the risk of SDR and consider alternative therapies for high-risk individuals, ultimately enhancing patient safety, increasing treatment success rates, and reducing the healthcare burden associated with severe drug reactions.
Graphical Abstract
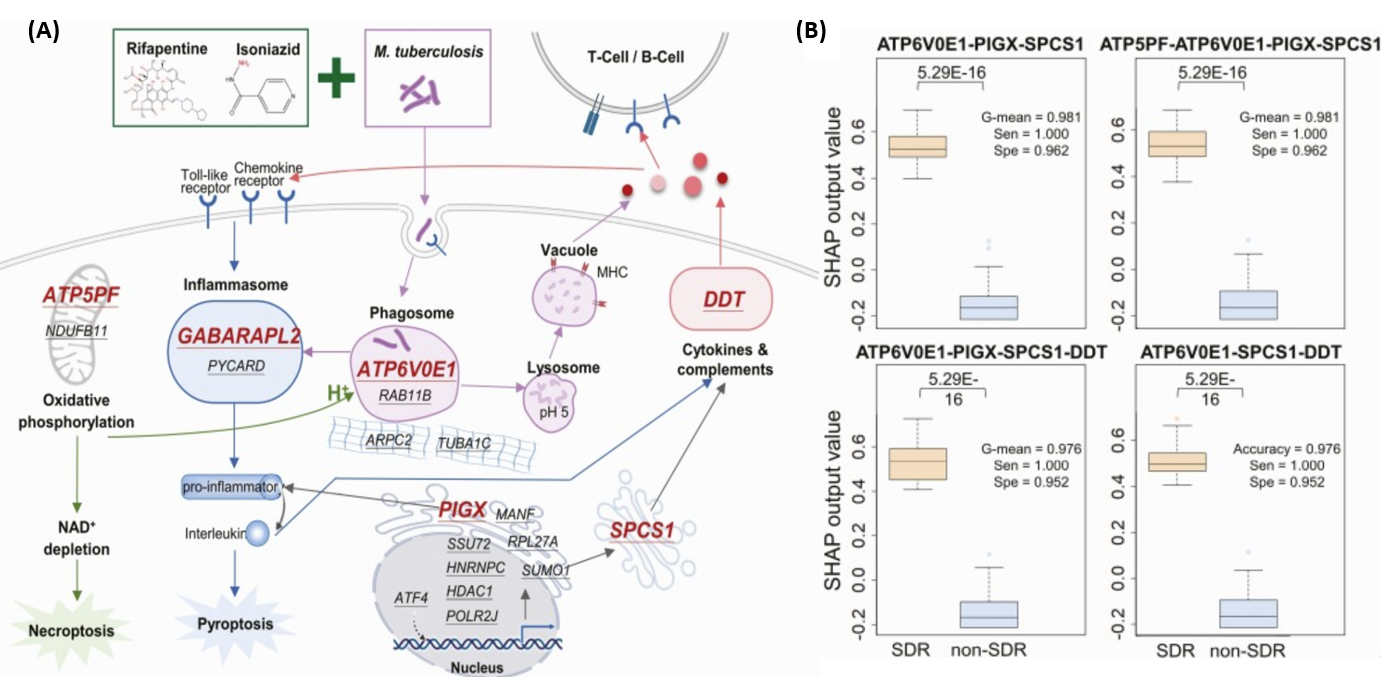
(A) Therapy–biomarker pathways for illustrating potential genes associated with SDR development under 3HP treatment.
For better visualization, the 19 potential biomarkers are underlined with the 6 selected genes via gene expression levels
marked in red.
(B)Box plot of the SHAP output values of the 4 best performing models using 6 selected genes in SDR (orange) and non-SDR
(steel blue) training samples.
Abbreviations: G-mean, geometric mean; RF, random forest; SDR, systemic drug reaction; Sen, sensitivity; SHAP, SHapley Additive exPlanations; Spe, specificity.
Application and Highlights:
Highlights:
1.This study combines clinical side effect phenotypes with genotypes, using biological functional analysis and interpretable
AI models to establish a simple clinical gene-based predictive model.
2.The genetic test model evaluates the risk of 3HP related systemic adverse reactions before LTBI treatment, allowing for
more personalized treatment plans.
Applications:
1.The methodology of combining AI technology with genetic analysis used in this study can be applied to develop risk
prediction models for severe adverse drug reactions in other medications.
2.By identifying high-risk individuals for severe drug reactions, healthcare costs and hospitalization rates associated with
severe drug reactions can be reduced, leading to more efficient allocation of medical resources.
Research Team Members:
1. Hung Ling Huang
https://wac.kmu.edu.tw/qur/profiles.php?id=990325
2.Jann Yuan Wang
https://www.ntuh.gov.tw/Med/Vcard.action?q_type=A04&q_itemCode=136
3.Jinn Moon Yang
https://dbt.nycu.edu.tw/faculty/yang-jinn-moon/
4.Meng Hsuan Cheng
https://wac.kmu.edu.tw/qur/profiles.php?id=880298
5.Po Liang Lu
https://wac.kmu.edu.tw/qur/profiles.php?id=830166
6.Inn Wen Chong
https://wac.kmu.edu.tw/qur/profiles.php?id=730138
7.Meng Rui Lee
https://www.hch.gov.tw/?aid=51&pid=3&page_name=detail&iid=5
8. Chih Hsin Lee
https://glb2sys.tmu.edu.tw:8020/TchInfo_Public/TchInfo.aspx?f=Tch&key=MTAzMTA3&key1=
Representative Department:
Division of Pulmonary and Critical Care Medicine, Department of internal medicine, Kaohsiung Medical University Hospital, Kaohsiung, Taiwan、Department of internal medicine, National Taiwan University Hospital、Institute of Bioinformatics and Systems Biology, National Yang Ming Chiao Tung University, Hsinchu, Taiwan、Division of Infectious Disease, Department of internal medicine, Kaohsiung Medical University Hospital, Kaohsiung, Taiwan、Division of Pulmonary Medicine and Pulmonary Research Center, Wanfang Hospital, Taipei Medical University, Taipei, Taiwan
Introduction of Research Team: The research team is composed of pulmonary and infectious disease physicians responsible for patient diagnosis, treatment, and clinical sample collection from Kaohsiung Medical University Hospital, National Taiwan University Hospital, and Taipei Municipal Wanfang Hospital, along with Professor Jinn-Moon Yang’s Drug Design and Systems Biology Laboratory from the Department of Biotechnology at National Yang Ming Chiao Tung University. The central philosophy of our team is to challenge the unknown, develop clinically applicable diagnostics, and pursue personalized precision medicine.
Contact Email: 990325kmuh@gmail.com
Publication: Clin Infect Dis. 2022 Sep 14;75(5):743-752. doi:10.1093/cid/ciac003.
Full-Text Article: https://www.ncbi.nlm.nih.gov/pmc/articles/PMC9477448/